This weekend, we started listening to the Acquired podcast's deep dive on Epic Systems, and it was, in a word, epic.
We're talking about the little-known healthcare tech giant behind the MyChart portal that 191M Americans use. Get this:
- In 47 years, Epic has never lost a customer (okay, one left for 6 months then crawled back).
- Founder Judy Faulkner bootstrapped the entire thing with just a $70K loan and a $70K friends and family round—and zero VC cash.
- They famously don't do acquisitions, barely invest in sales, and have never planned to go public.
- While Forbes has estimated Judy Faulkner's net worth in the billions, some industry analysts speculate the private company could actually be worth up to $100B.
$100B if it ever sold, that is (which Judy's trust ensures it won't). For now, it’s a $5.7B revenue behemoth quietly running the backend of most US hospitals.
Seriously, their Wisconsin campus has an 11,400-seat underground auditorium. Because, why not?
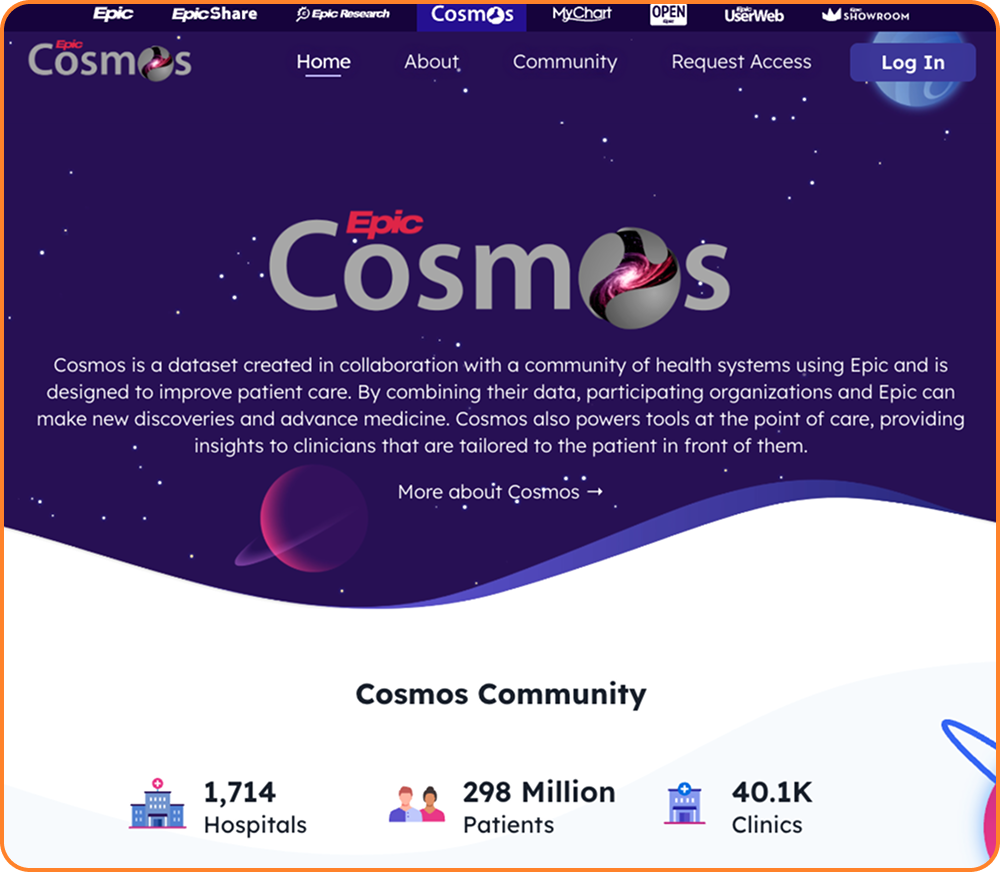
But while Epic holds down the hospital fort, AI is chipping away at healthcare's other massive headaches: confusing benefits and terrifying diagnoses. Two recent funding rounds caught our eye:
First, Healthee makes benefits less painful. Navigating your health insurance feels like a full-time job, right? Healthee just snagged $50M in an oversubscribed Series B (meaning investors were chasing them) to fix that.
- What it does: It's an AI platform that acts like a super-smart HR assistant for employees. Ask it benefits questions, get help with open enrollment, even receive personalized nudges for preventative care.
- Why it matters: Employer healthcare costs are projected to hit $16K per employee next year. Yikes.
- Tools like Healthee aim to make that less painful for companies and less confusing for workers trying to figure out their copays.
- They've already rocketed past 15K customers, including big names like Instacart and SiriusXM.
And then there’s Craif, which spots cancer.. in your pee?! Okay, this ones a little weird, but bare with us. Japan's Craif raised $22M to bring its AI-powered, urine-based cancer detection test to the US. Their valuation? Just shy of $100M.
- How it works: Instead of blood, Craif analyzes microRNA (miRNA) in urine.
- These tiny molecules are apparently early warning flares sent out by cancer cells.
- AI helps spot patterns indicating seven different cancers (like pancreatic, lung, breast, ovarian) potentially years before symptoms show.
- Why this matters: Urine samples are less invasive than blood draws (duh) and might have fewer impurities, leading to clearer signals.
- Oh, and high compliance is key for early detection—people are just more likely to pee in a cup.
Craif is already used by 1K+ institutions in Japan and making revenue ($5M last year, targeting $15M). The new cash funds US expansion: aiming for US clinical trials complete by late 2026 and FDA approval as early as 2027.
Healthee is tackling the frustrating administrative layer between employers and employees, while Craif is going after the holy grail of early, non-invasive diagnostics. And both are betting heavily on AI to simplify, personalize, and potentially save lives (and money, obviously).
But AI's potential goes way deeper than just streamlining admin or improving diagnostics – it's starting to fundamentally change how we understand disease itself.
The biggest healthcare breakthrough will be AI connecting molecular biology to clinical care. Not just making current processes efficient but accelerating our understanding of disease mechanisms human researchers might never uncover alone from combining AI with massive, unified health datasets…like, idk, Epic's MyChart??
Case in point: AI just cracked a piece of the Alzheimer's puzzle.
Scientists at UC San Diego used AI– specifically the protein-folding prediction kind, like AlphaFold, not a language model – to visualize the 3D structure of a protein called PHGDH. This protein was already known as an Alzheimer's biomarker, but the AI revealed something completely hidden in its sequence: a substructure that strikingly resembled a DNA-binding domain.
Turns out, this hidden function allows PHGDH to mess with how brain cells turn genes on and off, triggering a pathway that leads to Alzheimer's pathology.
As study author Sheng Zhong put it, “It really demanded modern AI to formulate the three-dimensional structure very precisely to make this discovery.” Even better, using AI modeling again, they identified an existing small molecule (NCT-503) that could bind to this specific substructure, block its harmful moonlighting role (without disrupting its normal job), and significantly improve memory and anxiety in mouse models of Alzheimer's.
This kind of breakthrough – AI uncovering hidden biological mechanisms – got the gears turning over on Hacker News. Commenter jedberg noted how good AI/ML is at finding correlations in huge datasets that humans miss. They speculated: What if we could apply that AI power to to everyone's anonymized medical records?
Imagine AI spotting patterns like, hypothetically, "four years before presenting symptoms of pancreatic cancer, patients complain of increased nosebleeds." Having massive, unified health data could unlock cures and leading indicators for countless diseases.
And this theoretical power of massive data analysis? It crashes right back into Epic Systems. While the US system is fragmented, Epic already runs Cosmos, a real-world version of this concept. It's a colossal, anonymized database pooling clinical data from 295M patients and 15B patient encounters across their consenting hospital customers. It's specifically designed for large-scale analysis – finding patient "lookalikes" for rare diseases, tracking treatment outcomes, identifying drug interactions, and enabling exactly the kind of research the UCSD team did, but potentially at an even vaster scale using real-world clinical data. Cosmos is the closest thing we have today to that Hacker News "what-if" vision.
Now, a few caveats:
Cosmos primarily contains clinical data, not the deep molecular data (like full genomic sequences for most patients, detailed protein structures, cellular imaging) needed for the type of discovery made in the UCSD study (which relied on AI analyzing protein structure). AI needs the right kind of input data. You can't easily infer a hidden protein function solely from ICD codes and medication lists recorded in an EHR.
The real revolution happens when you can link the clinical data in Cosmos (with appropriate privacy safeguards) to other datasets, like genomics databases, biobanks with tissue samples, proteomics data, etc. AI could then bridge the gap, connecting the clinical observations in Cosmos to the underlying molecular data from other sources to uncover novel mechanisms.
Quick, somebody tell Judy!
Actually, Epic's Cosmos database IS actively being used for this kinds of advanced analysis, although the primary focus seems to be on clinical patterns and outcomes derived from EHR data.
Here's how it works:
- Finding Complex Clinical Patterns & Generating Hypotheses:
- Cosmos is explicitly used for research to understand disease causes, treatment patterns, and prevention (paper).
- Researchers use it to explore connections, like the link between alopecia areata and thyroid dysfunction in patients with Down syndrome, using its large sample size which is crucial for studying rarer conditions.
- It helps characterize diseases, care patterns, and outcomes, as seen in studies on maternal/neonatal outcomes related to COVID, fentanyl testing rates, and infection rates in patients with alcohol use disorder.
- And it's used for public health surveillance, like tracking COVID and mpox trends with the CDC.
- Predictive Modeling & Machine Learning:
- Cosmos is explicitly designed for and used in predictive modeling. For example, UCSF uses it for this purpose.
- Studies have used Cosmos data to train machine learning models (like logistic regression, LGBM, RNN) to predict outcomes, such as vision impairment in Multiple Sclerosis patients (paper).
- Hospitals are using predictive models developed with or validated by Cosmos data, like Epic's Deterioration Index, to identify at-risk patients and improve outcomes (e.g., reducing mortality).
- Epic itself is developing "Cosmo AI" solutions using Cosmos data. For example, Epic is developing AI-powered tools within MyChart and clinician workflows (like "Best Care Choices" and "Look-Alikes") that leverage insights derived from Cosmos data analysis.
So yeah, Cosmos is currently being used by researchers, health systems, and Epic itself for sophisticated analysis to uncover clinical patterns, improve care quality, support clinical decisions, and conduct large-scale research. Who coulda guessed the world's most comprehensive and successful health data company would have a couple AI deals already lined up!