When most people think about AI, their minds immediately go to ChatGPT. But it all started wayyyy before OpenAI.
1950. Alan Turing. University of Manchester.
The computer scientist first had the idea that a machine could mimic a human's behavior if given the right instructions.
This led to the Turing Test, in which a person tries to distinguish an AI’s speech from a human’s. When people today say that ChatGPT-4o passes the Turing Test, they’re saying it can mimic human-level intelligence.

6 years later, researchers gathered at Dartmouth and asked themselves a similar question: “Hey, how can we build machines that simulate humans?”
During the conference, this dude John McCarthy actually coined the term “artificial intelligence.” Legend.
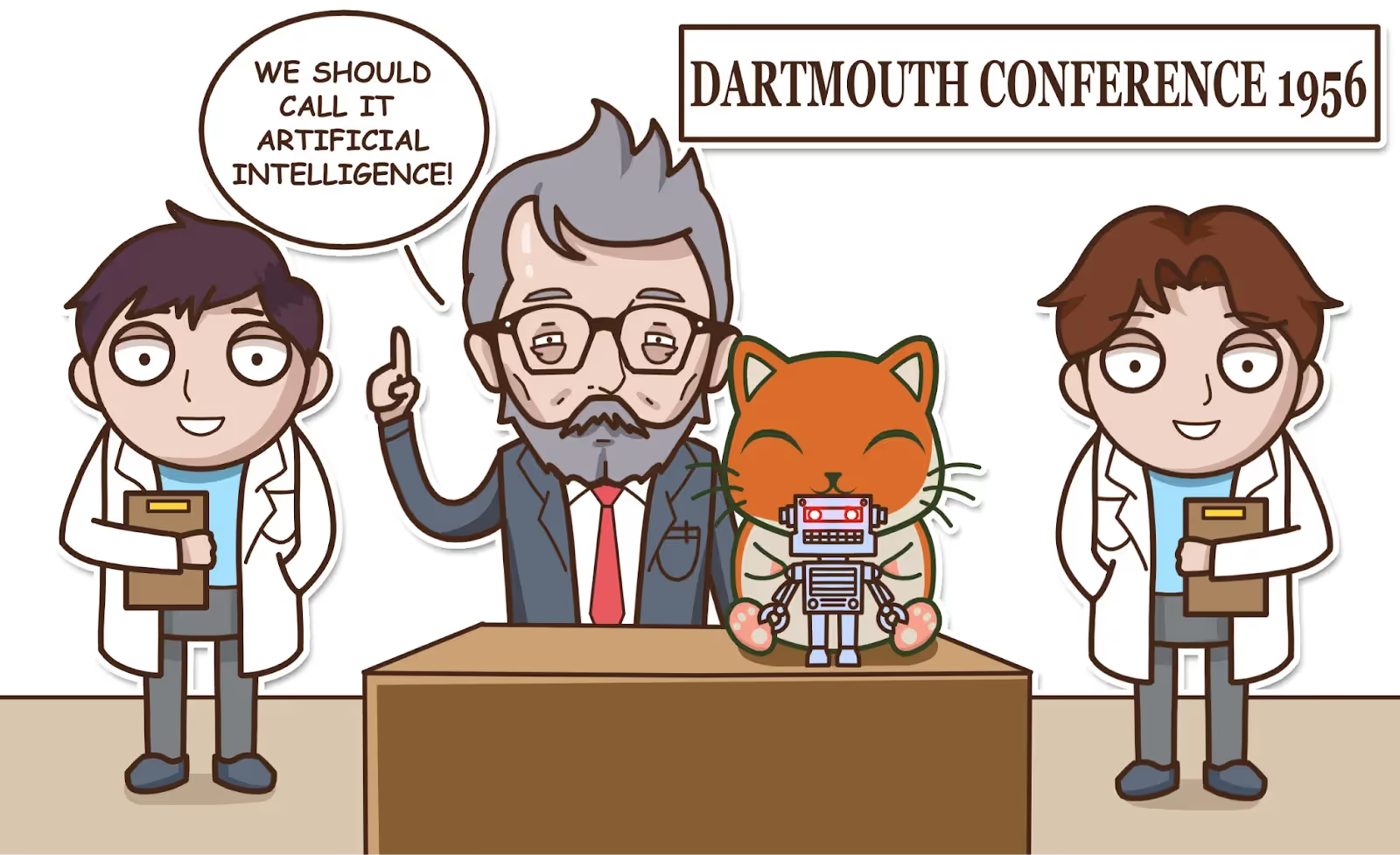
But 1900s-AI wasn’t the AI we think of today.
It was more like computers that followed a set of rules.
In one instance, in 1995, IBM (the Google of the 90s) launched a computer program called Deep Blue, which analyzed millions of chess moves to defeat the world champ Garry Kasparov.

BTW, this is the narrow AI we discussed yesterday—an AI that’s good at one thing!
But how the hell did we go from Deep Blue to something as advanced as ChatGPT???
Well, if a computer could predict and generate a chess move based on a set of rules, then shouldn’t it be able to predict and generate language based on a set of rules, too?
Enter language models.
These bad boys, called n-gram models back in the day, were all about predicting human sentences.
For example, with the phrase “Happy Birthday to _____,” a language model could guess “you.” Like a soulmate finishing your sentence!
But, like any relationship, these n-grams had their hang-ups.
When a language model predicts what comes next in a sentence, it juggles multiple possibilities (e.g., is it Happy Birthday to “you” or “John” or “them” or nobody?).
And when you go from predicting one sentence to one paragraph to one essay, the # of possibilities increases exponentially (think from 10 to 10,000 to 10,000,000).

And n-grams were only good at juggling a few possibilities at a time, not 10,000. This limited their ability to predict more than just a sentence, often resulting in disjointed and incoherent text over longer paragraphs.
Plus, The computers back then weren’t strong enough to handle millions, billions, or even trillions of combinations of possibilities at once.
2017. Google. “Attention is All You Need.”
This groundbreaking research paper introduced a way to break through this barrier: the Transformer.
If n-grams were a 1965 Ford Mustang, then a Transformer is a Tesla.
The Transformer allowed a computer to eat exponentially more language data—it was like teaching a kid to go from sounding out words to devouring entire books.

When you can handle more language data, you can handle more language possibilities, and you can generate entirely new content that makes sense when you read it.
Hold on. Generate. Reminds me of generative.
Oh, sh*t!
GPT = Generative. Pretrained. Transformer.
ChatG.P.T.!
And that’s how the world changed, folks.
Tomorrow, we’re diving into what this transformed world looks like today.
See you cool cats later,
Noah